Moisture Sensor Data Processing: From Raw Signal to Accurate Measurements and Classification
Soil moisture sensors are essential tools for monitoring agricultural and environmental conditions. However, their accuracy can be compromised by various factors. While the impact of each factor may be small, collectively, they can significantly degrade the reliability of sensor measurements.
Unraveling the Data Processing Pipeline: A Step-by-Step Guide
The figure below outlines the steps involved in converting a raw moisture sensor (soil or soilless) signal into usable percentage measurements. The data processing pipeline includes several key steps: temperature compensation to account for temperature-induced variations, noise reduction to eliminate unwanted signal fluctuations, and baseline correction to establish a reference point for dry conditions.
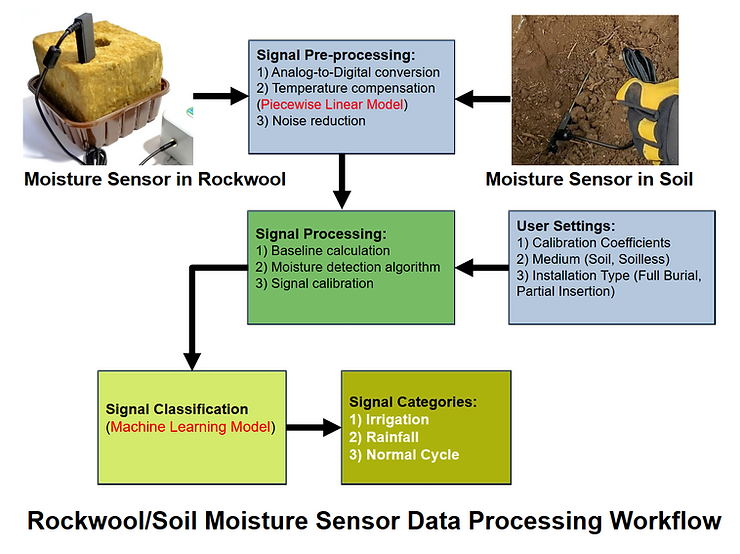
By applying these techniques, the processed data provides a clear representation of the moisture level, facilitating identification and analysis. While signal classification can be a useful tool, its reliability heavily depends on data quality and site-specific conditions, making it an optional step.
The sensor (APAS T1) measures medium water content by detecting changes in its surface's dielectric constant. The sensor's analog output provides a continuous signal representing the moisture level.
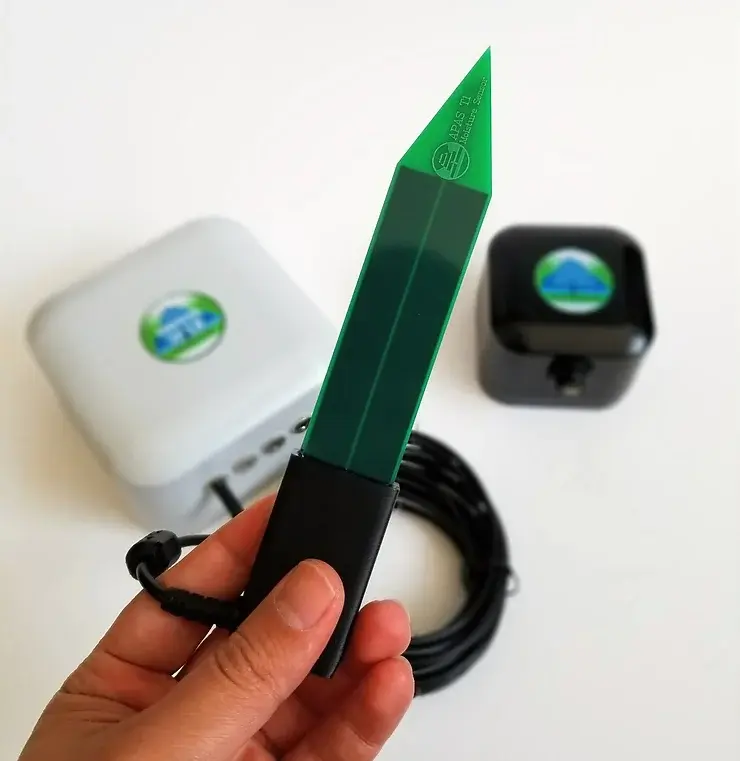
Additionally, the digital temperature output is used to compensate for medium temperature variations. However, the raw analog sensor signal is susceptible to noise and temperature variations, making it difficult to accurately correlate sensor readings with actual moisture levels.
Without proper processing, time-series data can be misleading, as it may be difficult to distinguish true moisture signals from background noise.
Signal Pre-processing
This involves initial steps like analog-to-digital conversion, temperature compensation, and noise reduction. The goal is to clean and prepare the raw sensor data for further analysis.
Signal Processing
This includes steps such as baseline calculation, feature extraction, and normalization. These steps consider user settings, including calibration coefficients, medium type (soil or soilless), and installation type (full burial or partial insertion).
By transforming the raw data into a suitable format, these steps prepare the data for the subsequent machine learning algorithm.
Signal Classification
The processed data is fed into a machine learning model, which classifies the signal into different categories (e.g., rainfall, irrigation, normal cycle).
The Complex Relationship Between Soil Moisture and Temperature: A Novel Approach
One of my most important research findings is the intricate non-linear relationship between soil moisture and temperature. Through extensive data analysis and experimentation, I discovered that the relationship between soil moisture readings and temperature is not a simple linear function, but rather exhibits distinct phases, each with its own linear relationship.
To address this complexity, I developed a novel piecewise linear regression model. This model accurately accounts for the varying temperature sensitivity across different soil moisture ranges, resulting in significantly improved accuracy and reliability.
I hope to share more details on the piecewise model in a future article.
Comments
Post a Comment